Introduction
Data science is the concept of using algorithms and systemic processes to collect, analyze, and organize data in order to derive patterns and trends. This can then be applied within the contextual understanding and industry parameters to determine optimal mechanisms and solutions to business challenges. Supply chain management and logistics benefits significantly from data science as multiple points of shipments, transfer, routing, and delivery can be processed and optimized.
The complexity and extensive amount of data generated by the supply chains of global corporations create a demand for competent methods of data analysis. Data science in supply chains holds tremendous importance and will continue to serve a greater role in the business prospects of developing logistics systems as the volume and frequency of supply chain transportation will increase.
Background
Supply chain logistics consist of numerous parts of the larger system that operate simultaneously to achieve a ceaseless sequence of production and distribution of products and services in any given company or industry. The modern supply chains encompass multiple interconnected links such as manufacturing facilities, GPS trackers on transportation vehicles, shipment and sales data, and sensor networks which altogether produce a significant amount of autonomous data.
Data science becomes vital for supply chain management by providing technology, tools, and opportunity to competently organize this data and analyze it for the purpose of operational optimization. Academia and business practitioners agree that the overwhelming amount of data produced promotes the use of big data analytics to gain a better understanding and uncover hidden values. In turn, this can be utilized by the firm to improve customer service, expand into new markets, increase its sales, and optimize supply chain management (Tiwari, Wee, & Daryanto, 2018).
The volume and complexity of supply chains in the modern global economy require similarly intricate analysis tools for in-depth analysis. Data science provides the capability to develop deep insights into patterns and provide a competitive advantage or forecasting models which are invaluable in supply chain management. This directly impacts areas of supply chain logistics such as stockouts, production, obsolescence, and promotion strategy (Lengacher, 2018).
Data science directly ties into the concept of predictive analytics in the supply chain by significantly enhancing it through its forecasting properties. This leads to the supply chain process becoming more reliable, efficient, and reduces waste, all of which lower long-term costs. Advanced analytics offered by data science have improved capabilities in forecasting and risk assessment.
The management of supply chain performances is vital for modern businesses due to regulatory barriers, the complexity of globalization, volatility of supply and demand, and an increased risk of production due to dependence on suppliers. Supply chain produces both structured and unstructured data. Structured data points include business systems information such as transactional data about purchases, production orders, and sales. Meanwhile, unstructured data can include aspects such as price management, supply chain planning, risk management, and transportation planning. Furthermore, complex and difficult to measure factors that encompass customer sentiments and demands, or risk and opportunities are inherently part of a “live” and unstructured supply chain data (Thalbauer, 2017).
Machine Learning and Other Data Science Trends
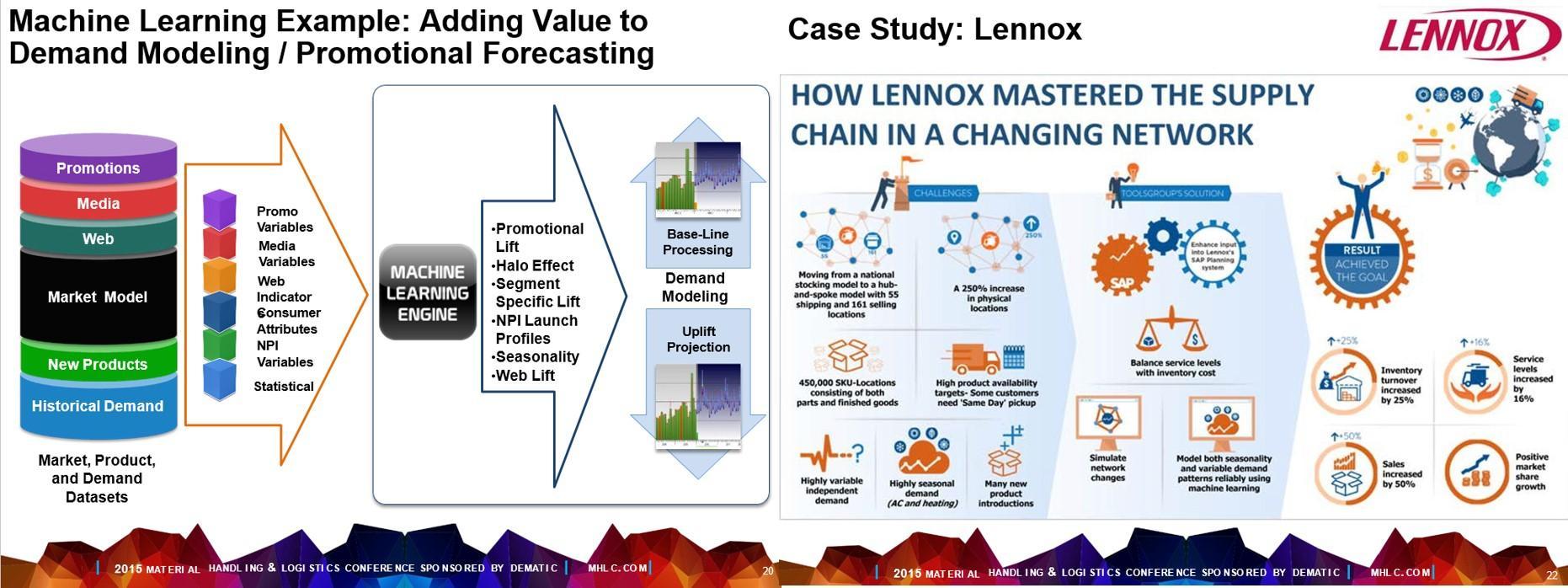
The central principle of introducing data science into supply chains is to digitize them in order to create “sentient” supply chains. These complex algorithms maintain the ability to collect data, process and understand it to extract value. It is then visualized and communicated in a streamlined manner. The scarce factor in many digitized supply chain systems is the lack of value extraction and presentation. A successful supply chain is inherently sentient, able to process and understand live data and demonstrate a level of awareness and responsiveness.
A data system of this extent is capable of intelligently transform mass data into optimal insights and make proper decisions which would allow it or a human to adequately respond to changing conditions. A sentient supply chain system is based on the steps of sense-decide-respond that leverage data science for informed decision-making processes for the company (Manenti, 2017). A sentient supply chain is dependent on the machine learning capabilities of the data science processes and systems.
Machine learning algorithms analyze tremendous amounts of diverse data which can improve forecasting accuracy, a critical challenge in supply chain logistics. Future demands for production are determined through advanced simulation modeling that accounts for moving averages and statistical analysis. The figure above provides an example of how Lennox radically transformed its supply chain by introducing machine learning; thus, not only improving forecasting but optimizing material handling and logistics conference.
It is evident that the machine learning engine is able to use tools such as baseline processing and uplift project. It was able to balance service levels with inventory costs, simulate network changes, and model seasonal and variable demand. It led to increased inventory turnover, service levels, and sales which positively reflected on market share growth.
Machine and deep learning of data science systems are efficient at pattern recognition, both visual and statistical. In the supply chain, this contributes to several distinct aspects. The algorithm can determine horizontal collaboration synergies amongst shipper networks. This can be beneficial in reducing freight costs, improving supplier performance while minimizing risk. Furthermore, this technology can provide visual pattern recognition that is beneficial for physical inspection and maintenance.
For example, automated quality inspection at logistics hubs can isolate damaged shipments and provide recommendations to either repair or discard the asset in a manner which is least costly to the company. Meanwhile, the tracking capabilities will help to determine if a specific route in the supply chain is causing excessive amounts of damage to shipments, thus increasing the risk. Machine learning provides deep insights into management performance that technologies beforehand could not provide. This includes supervised and reinforced learning that continuously identifies key influences in the supply chain link following algorithmic logic across large-scale enterprises (Columbus, 2018a).
Current trends for data science in supply chains are focused on forecasting as a tool to a pragmatic approach to demand and sales. A complex machine learning is capable of identifying causal factors of demand to provide more accurate forecasts for sales of unreleased products. However, data science is also helpful in enhancing the day-to-day operations of supply chains. This includes prolonging operational capacity and life of vital assets such as machinery, storage, and transportation. Overall supplier quality management and compliance are tracked more efficiently, which contributes to the manufacturing of in-house products as outside components are delivered promptly. Production planning and scheduling are optimized for effective workflows and removal of possible constraints to the process.
Data science analytics in supply chains is considered both a science and art by professional industries. It is constantly evolving to take advantage of any opportunities and avoid unnecessary costs or bottlenecks in manufacturing and distribution. The highest ranked uses of supply chain analytics amongst industry leaders in the coming years are to optimize producing and sourcing to reduce landed costs as well as analyze product cost variances (Columbus, 2018b). The trend for supply chains will focus on digitizing all processes and developing strategies for integration of data science and making improvements within company logistics.
Directions for Future Research
Data science and big data are a relatively recent introduction to supply chain logistics which creates extensive possibilities for future research and integration. One of the primary aspects of research that is demanded by the industry is the analytical capability to predict consumer behavior which is integral to supply chain logistics in contexts of product innovation, manufacturing, and distribution. Furthermore, data science can be used for inventory planning, facility layout design, and vehicle routing challenges. More complex aspects such as minimizing environmental uncertainties is also a potential expansion for data science research (Wamba, Gunasekaran, Papadopoulos, & Ngai, 2018).
A variety of frameworks exist for measuring data supply chains. However, there is no currently a unified methodology in data management that links strategy and operations in the context of supply chain analytics. Empirically linking roles of big data business analytics and supply chain analytics is critical to success in the industry. Research in descriptive analytics and connecting categories that fit within supply chain strategy and operations are a primary focus. A framework should be developed with methodologies to collect, analyze and distribute information amongst strategy and operations of supply chains to ensure sustainability (Wang, Gunasekaran, Ngai, & Papadopoulous, 2016).
Another avenue for future research is the implementation of data science tools and methods in business value realization at the level of supply chain management. There is an inherent gap between the analyzed big data and what humans can derive from it. Technologies and tools must be developed for visualization and access to the enormous amounts of data so that proper solutions can be integrated into supply chain management.
This can consist impact of data science on local portions of the supply chain, long-term IT expenditures, and overall ability to enhance efficiency for aspects such bottlenecks, predictive maintenance, and quality control (Wamba & Akter, 2016). Future research should pose the vital question of whether business models and supply chain logistics going forward should be implemented and expanded around the presumption that data science will be utilized. Furthermore, evaluating the impact of data science on traditional supply chains in various industries should be considered.
Conclusion
In the modern economy and business, supply chain management and data science are inherently interconnected. The success of a supply chain to adapt to the realities of risk and ever-changing economic environment depends on the ability of data science systems to collect, analyze, and produce insight on its operation. The integral capabilities that data science provides to enhance and improve supply chain operations are unarguable. This is possible with the capabilities of machine learning which revolutionizes the fundamental components of supply chain management through complex algorithmic analysis. Future directions of research focus on adapting data science into specific links of the supply chain system and optimizing it for business value realization.
References
Columbus, L. (2018a). 10 ways machine learning is revolutionizing supply chain management. Forbes. Web.
Columbus, L. (2018b). Analytics will revolutionize supply chains in 2018. Forbes. Web.
Lengacher, D. (2018). NextGen supply chain: Data science comes to the supply chain. Supply Chain Management Review. Web.
Manenti, P. (2017). Data scientists leap into the supply chain spotlight. Web.
Thalbauer, H. (2017). Living the live supply chain: Why you need data scientists. Digitalist Magazine. Web.
Tiwari, S., Wee, H., & Daryanto, Y. (2018). Big data analytics in supply chain management between 2010 and 2016: Insights to industries. Computers & Industrial Engineering, 115, 319-330. Web.
Wamba, S. F., & Akter, S. (2016). Big data analytics for supply chain management: A literature review and research agenda. In J. Barjis, R. Pergle, & E. Babkin (Eds.), Enterprise and organizational modeling and simulation: 11th international workshop, EOMAS 2015, held at CAiSE 2015, Stockholm, Sweden, 2015, selected papers (pp. 61-74). Cham, Switzerland: Springer.
Wamba, S. F., Gunasekaran, A., Papadopoulos, T., & Ngai, E. (2018). Big data analytics in logistics and supply chain management. The International Journal of Logistics Management, 29(2), 478-484. Web.
Wang, G., Gunasekaran, A., Ngai, E. W., & Papadopoulos, T. (2016). Big data analytics in logistics and supply chain management: Certain investigations for research and applications. International Journal of Production Economics, 176, 98-110. Web.